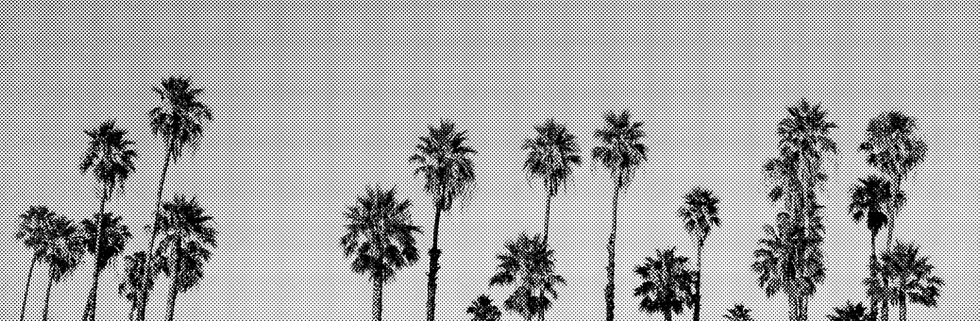
About
About

Our Data
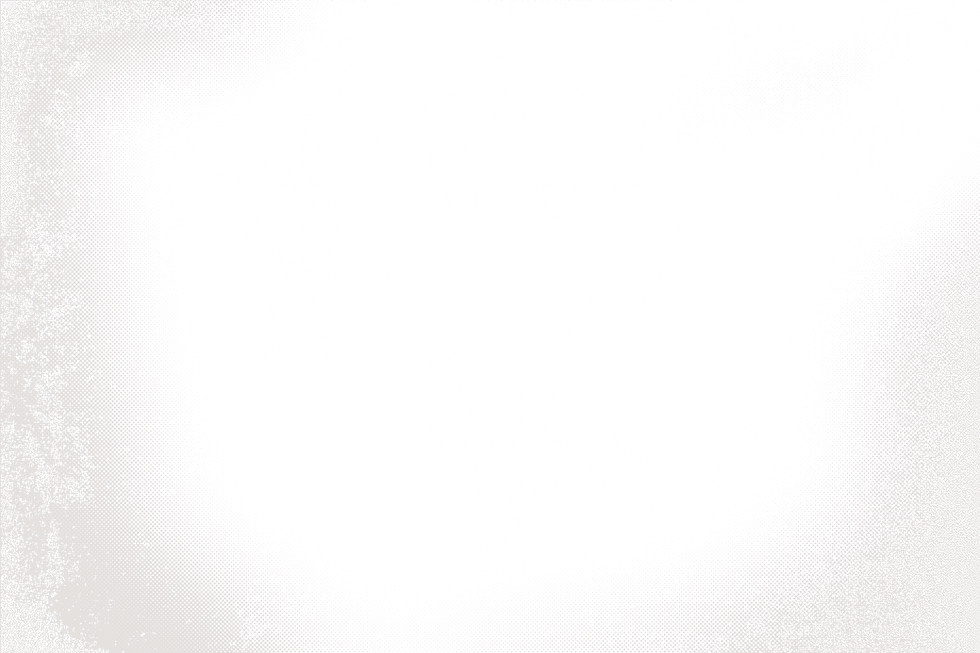
General
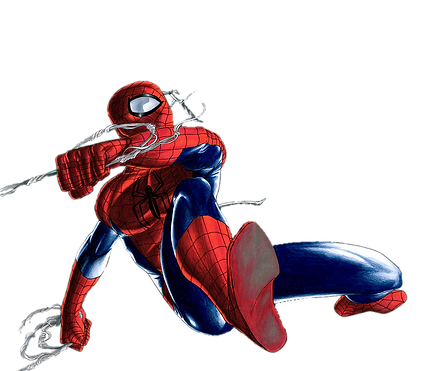
Sources
The Graphic Novel Corpus (GNC) served as our main source for determining our research questions with a total of 20 datasets, each serving a distinct component in regards to the overall collection of graphic novels. Out of the 20 datasets, only three were the most useful, the metadata of each book (graphic novel titles), author, and translations. The book dataset contained 219 graphic novels in total, each having their own “Book ID,” along with the average number of ratings, mentions, and date of primary publication. The author dataset provided variables such as the authors’ ethnicity, country of origin, country of residence, and gender. Lastly, the translation dataset presented various translations for each graphic novel title. After analyzing the data, we formulated possible research questions as a team, but further conducted secondary research thorough literature reviews in order to finalize our questions and strengthen our arguments as backed by the dataset. Additional support for our project came from academic journals that presented extensive information as supporting evidence for our research questions.
Image by MayanTimeGod on DeviantArt
Processing
To start, we took a dive on further investigating the GNC; as part of our data critique, it was important to note what was missing, how the data was gathered, and identifying any biases in our dataset. After taking account for certain biases found, we then proceeded to clean any possible errors in our data before choosing the datasets that we actually used for our research questions. As most of the data was well organized, we then took the next step of using Tableau for our data visualizations. Although the path we wanted to take with our research questions was not clear the first couple of weeks, using Tableau helped us analyze the various datasets and make correlations between them. As stated before, we used three datasets out of the 20 that were provided, by having the “Book ID” as the common factor between datasets, it was possible to then combine the datasets on Tableau. By being able to review the relationships between variables of the three datasets, such as ethnicity of authors, average ratings, and number translations as data visualizations, we were able to formulate our research questions with additional information from academic articles backing up our research questions.
Presentation
For the presentation of the website, we wanted to carry on the overall graphic novel theme as it is the main aspect of our research. As a result, we used common features that are associated with graphic novels. Features include characters from graphic novels, vibrant colors, and graphic novel text bubbles. It is also worth noting that we wanted to structure our website by starting off with our research questions, our findings from academic articles, visualizations, continuously building up leading up to the overall conclusion of the project. As previously mentioned, our data visualizations were conducted using tableau and our timeline was made possible by the Timeline JS tutorial.

Image by MayanTimeGod on DeviantArt
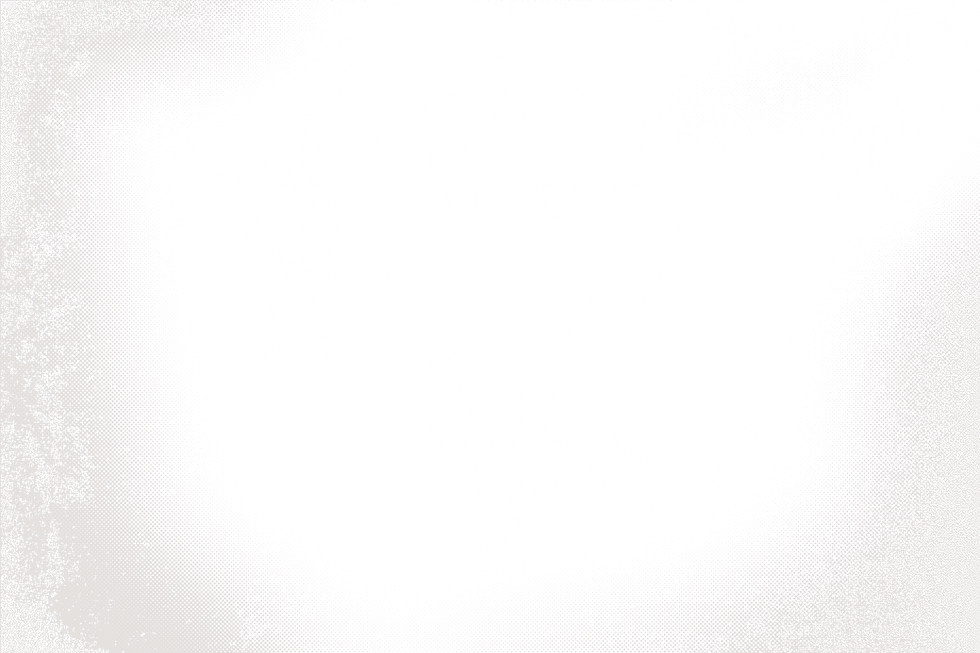
Data Critique
How was the information generated?

Image by MayanTimeGod on DeviantArt
The dataset was collected by different sources that showcase various aspects of graphic novels. The Graphic Narrative Corpus (GNC) was actually the first digital dataset for graphic novels created by Alexander Dunst, Rita Hartel, and Jochen Laudbrock. Resources used to create the datasets were from bibliographies, best selling graphic novels from Amazon. International award celebrations are also added to the datasets, categorizing prize winners related to comics and other forms of literature. Lastly, the information was gathered by reputable sources such as academic datasets, newspapers, and libraries compiled of graphic novels that are read by the adult audiences.
What are the original sources?
The original sources include around 240 different graphic novels of which 219 are actually accounted for in the dataset. Graphic novels, under the dataset creators definition is “book-length comics that exceed 64 pages in length, tell one continuous or closely related stories, are aimed primarily at an adult readership, and form one single volume or a limited series (such as a trilogy).” Although a definition always has to be established in order to categorize data, this definition severely narrows what can be included in the corpus. It excludes a wide range of very popular comics and other graphic novels that may have been shorter in length or a book that has a collection of shorter comics. Moreover, the graphic novels included are a combination of fiction and non-fiction. The authors admit that there is no way of knowing exactly how many graphic novels there are in existence, and therefore, they chose to draw from a very wide range of sources from many different authors and locations. The dataset includes the source of each graphic novel and where it was found. The graphic novels have titles ranging from A-Z and include a plethora of authors and genres, that are published sometime between the 70s and now. While there is a great variance among all the graphic novels, all their original languages are in English. However, about 40 of the novels come from Japan, France, Belgium, and Germany.
Who or what organization funded the creation of the dataset?
The project is created by Hybrid Narrativity (HN), which is a junior research group formed by graduate students from the University of Potsdam and the University of Paderborn that is focused on “digital and cognitive methods for researching graphic literature.” Leading the team is Alexander Dunst, a research assistant at the University of Potsdam and temporary advisor at the University of Paderborn. Other members of the Hybrid Narrativity include researchers from both institutions: Jochen Laurbrock, David Dubray, Rita Hartel, Oliver Moisich, Svitlana Zarytska, Volker Deppe, and Fabian Tegethoff. A majority of these researchers are well-versed in unique areas such as critical theory, cognitive psychology, computational statistics, and programming visualization software, among other things. Something we found particularly fascinating is that one of the goals of HN is the “collection of an empirical reference corpus of eye movement measures and development of corresponding analysis tools and measures in the form of an R package.” HN is continuously adding relevant data to this corpus and is actively conducting empirical research on graphic novels; for instance, HN presented their research at the European Society of Cognitive Psychology (ESCoP) in September 2019.
What information is left out of the spreadsheet?
Although information was gathered through sources such as Amazon bestseller lists, the GNC failed to provide other sources such as “Notable Awards” and “Prestige” datasets which rendered them impractical for use in our data formation. It is also worth noting the 219 novel dataset manifests no particular information on audience response, thoughts, and how they felt about the graphic novels. Furthermore, despite a plethora of data on the publication much of the information regarding publishers were not available. For example the demographics of publishers, this data set would have been greatly useful in our research to help understand more in depth the impact of minorities. We understand there is an under representation in the world of graphic novels regarding ethnicities. This leaves many open questions, such as: What about Black/Hispanic/Asian women in comparison to white women, and what about black/hispanic/asian men in comparison to white men? How is this reflected through various publishers? In regards to content, surveying some of the academic articles, such as “A Content-Analysis of Race, Gender, and Class in American Comic Book.” The article provides extensive research of 28 comics analyzing each frame of every comic, collecting data of how many protagonists were there, their race, economic status, and gender; the same collection of data was done for antagonists. This was not provided in our dataset.
What are the ideological effects?
As our data sets were collected by many reputable sources, such as graduate students from major Universities. We also had 20 datasets ranging from authors to translations, however the dataset provided did not allow us many options to formulate many collations. Our research was based on 219 graphic novels which is a very limited scope of the vast amount of graphic novels available to the public. Since not all the graphics novels are included in our data set we can assume that the results are most likely skewed. We also saw that some of the data was missing in the dataset. One example would be the ethnicity of authors. While the dataset included 249 authors, many of the ethnicity columns for the authors were marked unknown/null. Discrepancy such as this made it difficult to justify a graphic representation since the unknown/null variables were so high within the minority only chart (excluding whites). However when white authors are included we can clearly see that they are dominating the amount of novels they have published. Nonetheless, we were able to correlate a few graphs based on Geographical location, ethnicity, and the year the novels were published.
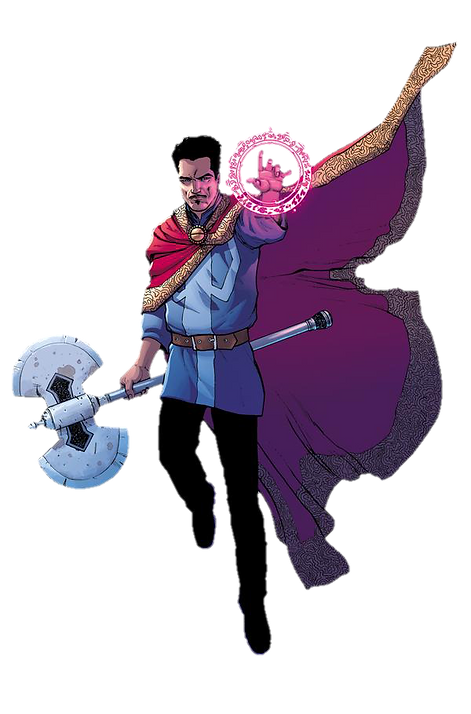
Image by MayanTimeGod on DeviantArt
Acknowledgements
We would like to express our thanks to Professor Sanders and TA Ruth for guiding us through this project. We are grateful for your knowledgeable insights, constant communication, and unwavering support as we worked to construct a meaningful narrative from our dataset— allowing us to personally discover the value of humanistic research.